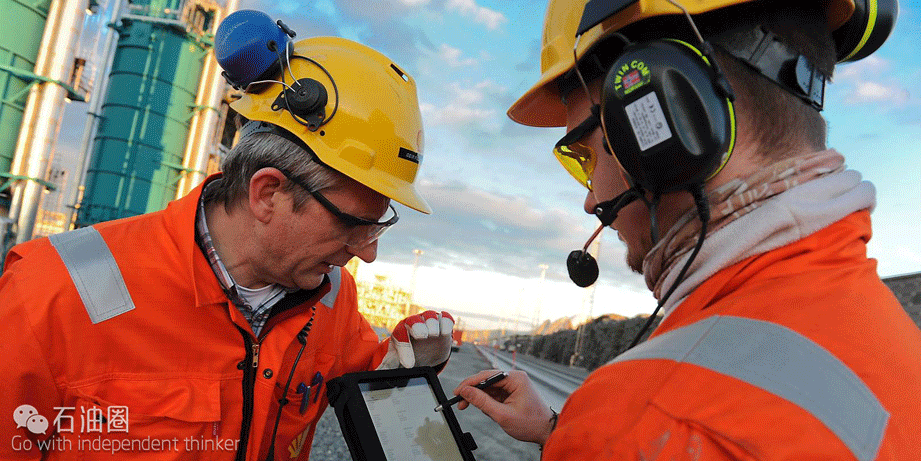
油气行业曾经是数字化变革的领先者,早在多年之前就提出了“数字油田”的概念。然而近年来,随着大数据技术的不断发展,油气行业将迎来新一轮的数字化变革。究竟我们需要什么样的大数据?本文也许会给你一些启发。
油气行业曾一度处于数字化技术应用的最前沿。大约50年前,油气行业首先采用数字分布控制系统对炼油厂和其他下游设施进行控制。此后,随着数字油田概念的发展,油气行业率先采用地震资料数字处理方法表示沉积物和储层,进行资源开采方案的优化、油井运行管理、油藏产量的提高以及避免损坏等。如今,油气行业仍将面对新的挑战:如何进行下一轮数字化变革,并彻底改变其资产性能管理的方式。
资产性能管理(APM)是对设备和生产进行实时监控器,并将一连串数据传输到中央设备,用大数据分析软件进行处理,找出最优的方案,是对硬件和软件的充分利用。这样可以帮助技术人员快速解决各种问题,节约大量时间,并减少因停机停工带来的成本浪费。
从某些方面来说,作为数字油田推广的先行者,油气行业在下一阶段的数字化变革中可能已经被甩在了后面。尽管石油公司在所有关键工序上都布置了传感器,且拥有多年的经验,但是在对行业物联网(IIoT)的认知方面,他们仍然无法满足客户的需求。类似地,许多行业内的技术人员对他们的数据分析能力非常自信,并指出他们从上世纪80年代就开始应用大数据,拥有10万多条这样的历史数据。
不幸的是,这些观点都忽略了数字化的真正核心,新一轮的数字化与大数据、预测分析、云技术和物联网有着密切的联系。这些技术的进步将共同成就油气行业的信息化,就如同16世纪末的工业化一样意义重大。与上世纪五六十年代蒸汽机的出现,电气化和自动化类似,信息化将是一个从量变到质变的过程。
油气行业的大数据不仅仅是“大量的数据”
随着物联网技术的应用越来越普遍,生产设施及其周围环境产生的各项数据呈指数增长。由于油气行业很早就开始记录生产过程的历史数据,因此其本身就积累了大量生产设施运行产生的数据。虽然数据量庞大,但是这些数据结构良好,可通过时间参数进行检索。从另一方面来说,大数据要复杂得多,它不仅包括结构化数据,还包括非结构化数据。例如,振动和温度记录系统产生的频谱数据、文本描述信息或者瞬时事件产生的无关信息。一般处理这些杂乱的数据要比处理时间序列数据所使用的外推或者内插方法复杂的多。
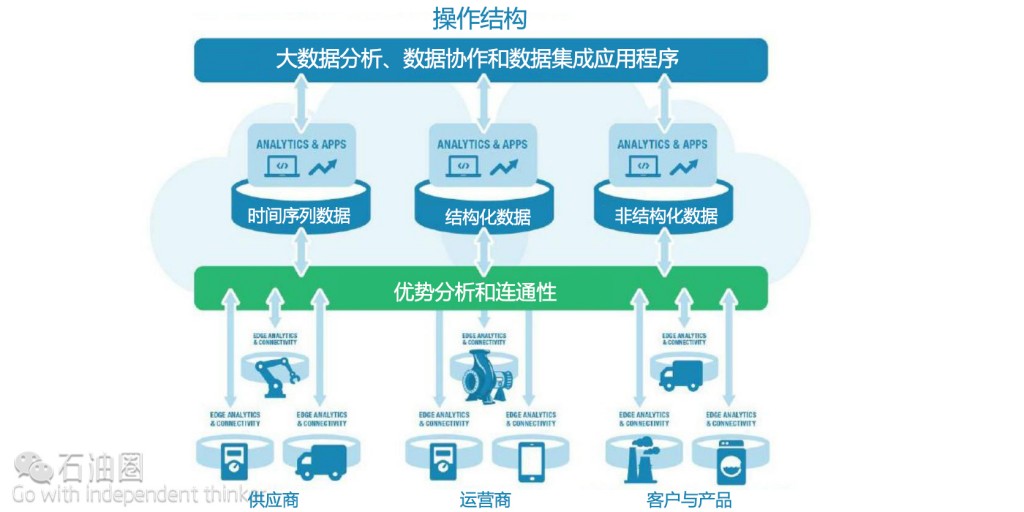
现有的基于模型的预测算法能够很好地处理时间序列数据,但是却无法处理“大数据”。这时候,云计算技术在处理大数据的容量和多样性方面就有了用武之地。但是对于更大容量的数据,则需要用到更高级的预测方法,这种方法拥有自我分析的能力,可以对新数据或者无法获取的数据加以利用。这种突破性技术对于油气行业加工过程和设备具有更强的动态分析能力。
下一代APM的关键是如何实现平台化
油气行业使用的设备价格昂贵,属于资本密集型行业,必须面对方方面面的风险。因此该行业所采用的任何系统都必须高度安全可靠且响应迅速。此外,油气行业的设备设施在不同地区,分布范围极其广泛,因而该行业对其所采用的计算平台拥有更高的要求,其云计算平台必须具备好的响应性、可靠性和安全性。Email服务器短时间内断开连接或者等待文字处理程序升级所造成的损失可能还在接受范围之内,但是油气行业的与之不同,如果是高压天然气压缩机断开连接所产生的不良后果是难以接受的,这有可能造成重大经济损失。
将完全迥异的应用程序整合为一个整体的做法所需的成本较高,对于面临巨大经济压力的石油行业来说不具有可行性,任何解决方案都必须拥有一个合理的成本。幸运的是,现在的技术已经可以满足这些要求。在过去5年的时间内,技术的进步已经克服了这些障碍。我们现在所需要做的就是给予用户信心,根据客户自身及其供应商的专业能力提出解决方案,将其数据转化成可执行信息。如今,预测分析已经可以缓解生产活动的复杂性给人们带来的困扰。对于油气行业来说,改变其固有文化迫在眉睫,第一个吃螃蟹的人,或许将获得巨大的优势。
油气行业的复兴取决于数字化变革成功与否
借助物联网技术和云计算平台,油气行业不仅可以提高效率、降低成本,而且还可以使产能再上一个台阶。行业的领导者们已经意识到,如何利用资产性能管理、物联网和业务统计调查,平衡企业的财务状况,控制运营风险并做出正确决策,会变得更加重要。当然,行业的监管力度和经济要求也将更加严峻。
作者/Dan Miklovic 译者/张强 编辑/Wang Yue
The oil and gas industry was, at one time, at the forefront of Digital Transformation in industry. Nearly 50 years ago, it was the first industry to use digital distributed control systems (DCS) to control refineries and other downstream plants. Then, with the deployment of the digital oil field concept, it became a leader in adopting digital representations of seismic data representing deposits and reserves. The challenge for the industry now is to implement the next wave of Digital Transformation and radically shift the way it approaches Asset Performance Management (APM).
In some respects, as an early adopter of digital field instrumentation, the oil and gas industry has set itself up to potentially be late to the table when it comes to the next phase of Digital Transformation. A common refrain LNS Research often hears when talking to clients about the Industrial Internet of Things (IIoT) is the retort that nothing is new and that “we have been doing this for ages” because their companies have had sensors in place on all of their key processes. Similarly, the industry also serves as the breeding ground for data historians, so many hold parallel perceptions about their standing within analytics capabilities, opining, “we have been doing Big Data since the 80s, because we have a historian with 100,000 tags.”
Unfortunately, this too misses the real heart of Digital Transformation today that results from Big Data and predictive analytics, cloud technology and the IIoT. The convergence of these technology advances today will cause the “informationization” of industry, with changes as profound as industrialization did in the late 1700s. There will be a step-change in productivity similar to those that occurred with the advent of steam power, electrification, and then automation in the 1950s and ‘60s.
Big Data in Oil & Gas Is About More Than Just “Lots of Data”
As IIoT technology becomes more prevalent, the amount and variety of information available about the condition of assets and the environment in which they are operating is growing exponentially. The industry, as an early adopter of process data historian technology, has been dealing with large volumes of time series information captured from the instrumentation that monitors and controls plant processes. While high in volume, this data is well structured, albeit simply indexed by time. Big Data, on the other hand, is far more varied, consisting of both structured and unstructured data. Examples include spectral data from vibration and thermography systems, textual descriptive information, and even potentially unrelated information about transitory events that may or may not be related. Making sense of this highly disjointed data is more complex than just extrapolating or interpolating from time series data that is highly correlated to the process itself.
Existing model-based predictive algorithms work well with historical time series data, but not so well with Big Data. Cloud technology certainly becomes beneficial in handling the volumes and variability of Big Data. But to an even larger degree, advanced predictive methods with self-learning capabilities to use new and previously unknown or unavailable data will truly be the breakthrough technology that provides better insight into process and asset dynamics.
A Platform Approach Provides Critical Capabilities for Next Generation APM
The oil and gas industry has to manage risks on many fronts, and the assets employed are expensive and capital intensive. Any systems supporting the industry must be highly reliable, responsive and secure. Add to this equation the fact that many of the assets are geographically widely dispersed, and it becomes obvious that the computational resources to support the industry have greater demands than typical commercial computing platforms. Responsiveness, reliability and security of cloud-based computing are essential. Losing connection to an email server for several seconds or waiting for a word processing document to update is acceptable; losing the connection to a high-pressure natural gas compressor to detect an impending problem isn’t. This is set against the backdrop of an industry that is facing some of the tightest economic margins ever. The ongoing cost of supporting a collection of disparate applications interfaced together isn’t feasible in the face of the current economic pressures in the industry. Any solution must not only be affordable to deploy but economical to support for the foreseeable future. Fortunately, technology today can meet these demands. Advances in technology over the last five years have overcome all of the hurdles. What is needed now is confidence in the user community to leverage their own and their suppliers’ domain expertise to build and deploy the solutions that can turn the accessible data into actionable information. Predictive analytics helps us overcome the complexity of production today. The only thing holding the oil and gas industry back is resistance to cultural change. The first players that make the leap stand to gain a significant competitive edge.
Digital Transformation Can Revitalize Oil & Gas
With a modern IIoT and cloud platform underlying the next generation of applications and analytics, the oil and gas industry can move beyond just doing the same thing faster or cheaper and adopt new levels of productivity. The ability to base asset care decisions on financial criteria balances against sustainability, safety and operational risk management goals addresses the top concerns that industry leaders have identified in LNS Research’s APM, IIoT, and Business Demographics surveys, namely surviving in a more regulated and economically challenged environment.
未经允许,不得转载本站任何文章:
-
- 甲基橙
-
石油圈认证作者
- 毕业于中国石油大学(华东),化学工程与技术专业,长期聚焦国内外油气行业最新最有价值的行业动态,具有数十万字行业观察编译经验,如需获取油气行业分析相关资料,请联系甲基橙(QQ:1085652456;微信18202257875)